Scale-Up vs. Scale-Out Storage: Why Choose?
There are good reasons to scale out storage and good reasons to scale up storage. The important thing is to apply the right model to the right storage use case.
June 28, 2019
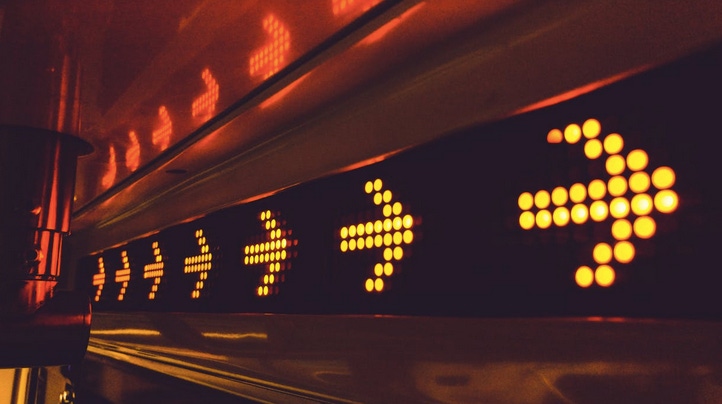
In recent years, scale-up storage has fallen a bit out of fashion, with many organizations preferring instead to focus on scale-out storage. In spite of the benefits provided by scale-out storage, scale up still has its place. The case can be made that the enterprise needs to be focusing on both scaling up and scaling out their on-premises storage.
For those who might not be familiar with the terms scale up and scale out, they refer to two different approaches to storage architecture. Scaling up basically means purchasing more capable hardware. This might mean, for example, trading in your existing storage array for a fancy new all-flash array. Scaling out is the exact opposite of scaling up. Rather than purchasing expensive new hardware, scaling out means achieving scale by investing in low cost, commodity hardware that can be used in a parallel architecture. This is the approach that the cloud providers use. These providers are able to scale their storage offerings to a massive level by leveraging low-cost hardware.
The question of whether it is better to scale up or to scale out has been asked many times. However, I’m not convinced that organizations need to take an either/or approach to scaling their storage. There are advantages and disadvantages to both types of scaling, and it is entirely possible that some data center functions will be well suited to using scale out storage, while others would benefit from scaling up.
So, with that said, let’s talk about some of the reasons why you might need to scale up your storage. Scaling up storage can be useful in situations in which a scale-out architecture simply does not deliver the required level of performance.
One of the reasons why a scale-out architecture works so well for hosting unstructured data is because the storage workload can be distributed across multiple storage nodes. However, this approach presents a couple of problems.
First, even if the scaled-out architecture provides the illusion of being a single, cohesive file system, there are node-level boundaries that exist. A large file, for example, typically cannot be split so that part of the file resides on one node while the rest of the file resides on another node (at least, not when a traditional file system is being used).
Herein lies the second problem. Because files cannot be split across nodes, individual files cannot benefit from the combined performance of multiple nodes. While this probably won’t present any problems for document files and other common file types, it could very well become a problem if someone were to try to host a database on the scaled-out storage. Remember, the individual nodes are commonly made up of commodity, X86 hardware, which is not exactly known for its performance. Scaling up can help you to overcome node-level performance limitations.
Another reason to consider scaling up your storage is because doing so may be good for the overall storage reliability. Although scaled-out storage architectures do tend to be reliable, they are also complex. Each one of the commodity machines that makes up the scaled out architecture could potentially become a point of failure. Now, granted, scaled-out storage is usually constructed in a way that provides resilience against a node failure, and that should theoretically make a scaled-out architecture more reliable than a scaled-up architecture. However, if a scaled-out architecture is made up of commodity X86 machines rather than enterprise-class hardware, then the individual nodes may lack the component-level redundancy that makes them less prone to failure.
One more reason why you may have to scale up your storage is because many scale-out solutions have a node limit or a capacity limit. This certainly isn’t true for every solution, but some limit you to a specific number of nodes and a certain maximum capacity. If you try scaling out and hit a node limit, then your only options will be to either silo the storage (by building another independent cluster) or to scale up the nodes within the existing cluster.
I’m not trying to make the case that scaling up your storage is somehow superior to a scale-out solution. As previously stated, both approaches have their place. There will likely be times when you will need to scale up your storage, and other times when you may be able to get away with scaling out. The important thing is to use the right model for the right use case.
About the Author(s)
You May Also Like