Tractica Report: Utilizing Deep Learning in Applications
Deep learning has been hyped by the business and technical press for years, often with relatively meager results that failed to live up to expectations. But over the past 18 months, the true power of deep learning has been realized, thanks to advances in hardware and algorithms that use pattern recognition applied in a continuous learning loop, enabling them to train themselves to perform tasks without requiring explicit programming code.
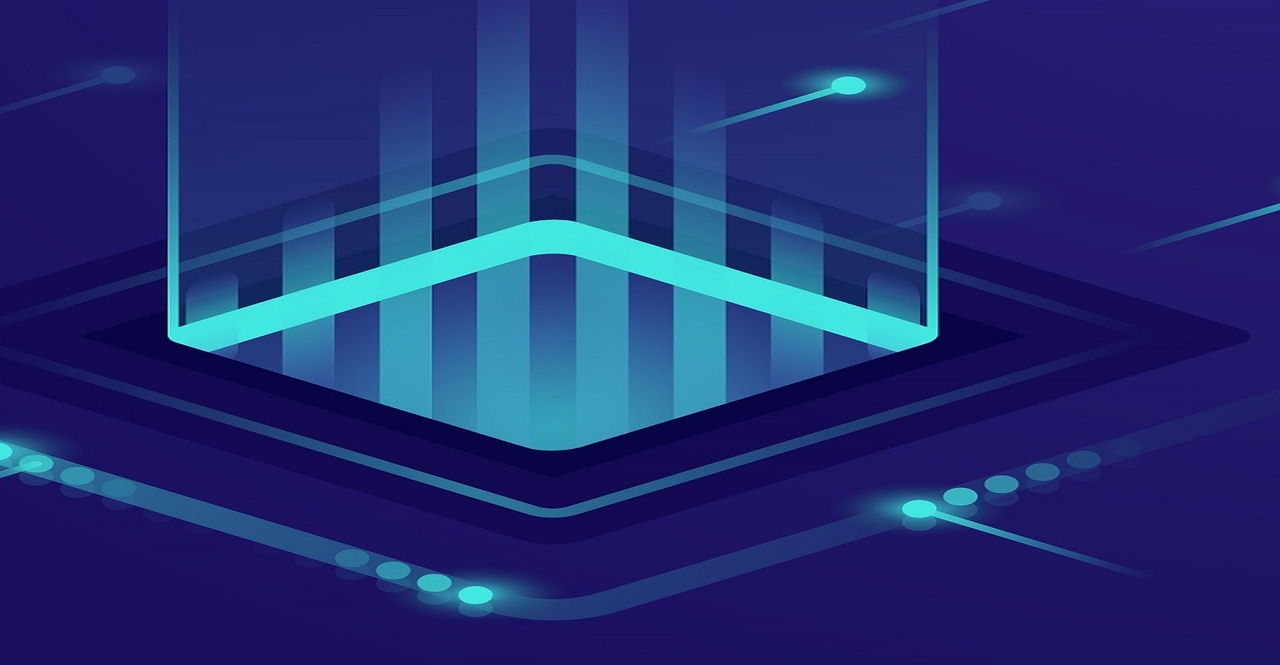
The promise of big data revolves around the ability to capture, process, and activate huge amounts of information within a reasonable amount of time. But as the amount of data that needs to be processed multiplies rapidly, the demand for even more powerful hardware and machine learning algorithms is driving the creation of more advanced deep learning software and frameworks. Ultimately, across a wide variety of industries, there is a growing push to create machines and systems that can accurately, quickly, and affordably mimic human intelligence.
As a subset of machine learning, deep learning is distinct in that it is composed of multi-layer (“deep”) neural networks wherein each layer of the network corresponds to different levels of abstraction. The true power of deep learning is seen when vast amounts of data are fed into a deep learning algorithm, and basic pattern recognition can be applied. This continuous learning loop enables algorithms to train themselves to perform tasks and adapt to new data. Deep learning’s key differentiating factor from other types of machine learning techniques is the ability to infer outcomes without explicit instructions, instead drawing from patterns within the data. This ability to learn on its own represents a key benefit for managing datasets that are simply too large to curate and manage manually.
Deep learning can be applied and is particularly well-suited to autonomously extracting nuance from any (structured or unstructured) dataset large enough to identify statistically significant patterns. Some of the most popular deep learning use cases in development today center around incremental, if very practical, advancements in areas such as image recognition, text analysis, product recommendations, fraud prevention, and content curation. The sheer power in deep learning, however, is likely to lead to the development of more powerful and disruptive applications of tomorrow, such as driverless cars, personalized education, and preventative healthcare.
Deep learning has been a key point of focus for many companies, given its potential to transform entire industries. Current leaders in the deep learning market include Google, Facebook, Microsoft, IBM, Amazon, Baidu, and others. The Chief Executive Officer (CEO) of Google, Sundar Pichai, described deep learning as “a core, transformative way by which we’re rethinking how we’re doing everything.”
Tractica research estimates deep learning to be the largest technology category in terms of revenue. Our research into AI has unearthed 125 use case categories in which deep learning constitutes a majority. Deep learning is often used to enhance or support other technologies, including computer vision, natural language processing (NLP), sensor data, and other technologies, allowing deep learning to touch an even greater variety of use cases and scenarios.
Tractica believes the opportunity for deep learning spans a wide range of industries and geographies and is particularly disruptive in highly domain-specific markets with high-volume data needs and ontologies, and those with growing applications for machine perception. Deep learning software revenue is estimated to grow from $3 billion in 2017 to $67.2 billion by 2025.
Despite the promise of deep learning, the approach faces a number of fundamental hurdles to adoption, which this report will outline. It also introduces new opportunities for improving accuracy, efficiency, costs, streamlined workflows, and more empirical decision-making.
Market Drivers
The ideas behind artificial intelligence (AI) and deep learning are decades old; humans have been trying to prescribe human-like qualities to machines since the 1950s. However, the rapid growth of deep learning today is due to the convergence of massive data generation; advancements in hardware speed; and improvements in machine learning algorithms. As a result, academia, startups, and enterprise stalwarts have each accelerated research efforts to develop deep learning platforms and enable new use commercially-focused use cases.
[This article is from research firm Tractica’s report on deep learning. View full report details.]
Tractica’s analysis includes a detailed assessment of business model impacts in both cost and resource efficiency gains, as well as new incremental revenue generation. To illustrate these potential business model impacts, Tractica assesses the benefits of deep learning in its ability to drive efficiencies in the form of speed, accuracy, agility, and access in the following areas:
Product development and improvement
Process optimization and functional workflows
Personalization and customer insight
Sales optimization
Innovation and longer-term strategy
Furthermore, deep learning offers a number of potential benefits to society. Tractica’s research explores both the benefits and the risks of AI and deep learning in society, exploring areas such as energy conservation, safety, public health, and others.
These market drivers have turned the heads of almost every large technology company, enterprise adopters across industries, herds of investors, countless “.ai” startups, and even governments and policymakers.
Market Barriers
Deep learning is likely to provide a number of benefits to commercial and public sector organizations. There are significant barriers to widespread adoption, which span both technological and non-technological areas of resistance. A key issue with deep learning (and AI, in general), is the significant gap between the high expectations of intelligence and the reality of current limitations of software and computing. This research explores the following areas that threaten the adoption of deep learning and AI in commercial environments:
Lack of accessibility and simplicity
Trust issues, including employee and consumer sensitivities
Ethical risks and unintended consequences
Job displacement and the transformation of work
Business challenges to obtaining data
Significant shortages in talent
Challenges of scale
Opacity in “explainability”
Technology Issues
This report examines deep learning architecture and technological considerations that will impact the market throughout the forecast period. Within these structures, Tractica explores a number of technical questions enterprises face:
Defining what deep learning is (and is not)
Contextualizing deep learning within broader machine learning and AI
Criteria for deep learning application
Application in conjunction with other technologies
Technical challenges of obtaining proper training data
Training and supervising deep learning models
Various hardware, firmware, and software configurations of deep learning
It should be noted that deep learning is still a nascent technology and is not a “magic bullet” technology that is suitable for all scenarios. It is characterized by its open-source development, and often the preferred path of implementation lies in cloud-based deployments. However, advancements in firmware-level machine learning and maturity in edge processing are shifting the narratives around data storage requirements, architectural development, and market access. These developments are outlined in this report as well and explored in greater depth in Tractica’s Deep Learning Chipsets report.
Use Cases for Deep Learning
Tractica’s research finds that use cases for deep learning span a wide range of 125 distinct use cases, touching at least 30 distinct industries. Some of the most common applications Tractica’s research surfaced across multiple industries include:
Voice/speech recognition
Static image recognition, classification, and tagging
Object detection, including navigation
Predictive maintenance
Trend identification and prediction (e.g., weather, demand, fraud, etc.)
Sensor data fusion
Within each of the use cases Tractica identified, countless sector-specific variations exist. As with most technological innovations, different industries will adopt pieces of this technology at varying paces. The qualitative portion of this report defines the more than 90 use cases for deep learning based on revenue, investment, and market activity. The quantitative forecast model accounts for all 125 use cases Tractica has identified in its ongoing coverage of the AI market.
Market Forecast
Tractica forecasts that annual software revenue for deep learning applications will increase from $3 billion worldwide in 2017 to $67.2 billion in 2025, representing a compound annual growth rate (CAGR) of 47.4%. Total annual revenue for deep learning software, services, and hardware will increase from $12 billion in 2017 to $283.8 billion in 2025, at a CAGR of 48.6%.
This report forecasts revenue across five world regions, 30 distinct industry verticals, and 125 use cases across enterprise, consumer, and defense markets. Some of these use cases include static image tagging, localization and mapping, predictive maintenance, and human emotion analysis, among many others.
Tractica also forecasts hardware and services revenue driven by deep learning. The hardware forecasts are further segmented into separate forecasts of the demand for central processing units (CPUs), field-programmable gate arrays (FPGAs), application-specific integrated circuits (ASICs), graphics processing units (GPUs), networking products, and data storage devices.
Deep learning applications will also generate significant demand for professional services, such as installation, training, customization, application integration, and maintenance, which are included in Tractica’s forecast. In addition to this market forecast, this Tractica report offers a comprehensive analysis and overview of the entire market opportunity and challenges for deep learning products and services.
About the Author(s)
You May Also Like