Enterprise AI: What to Watch for in 2020
DarwinAI’s Sheldon Fernandez shares insights into both past and future developments in enterprise AI and ML.
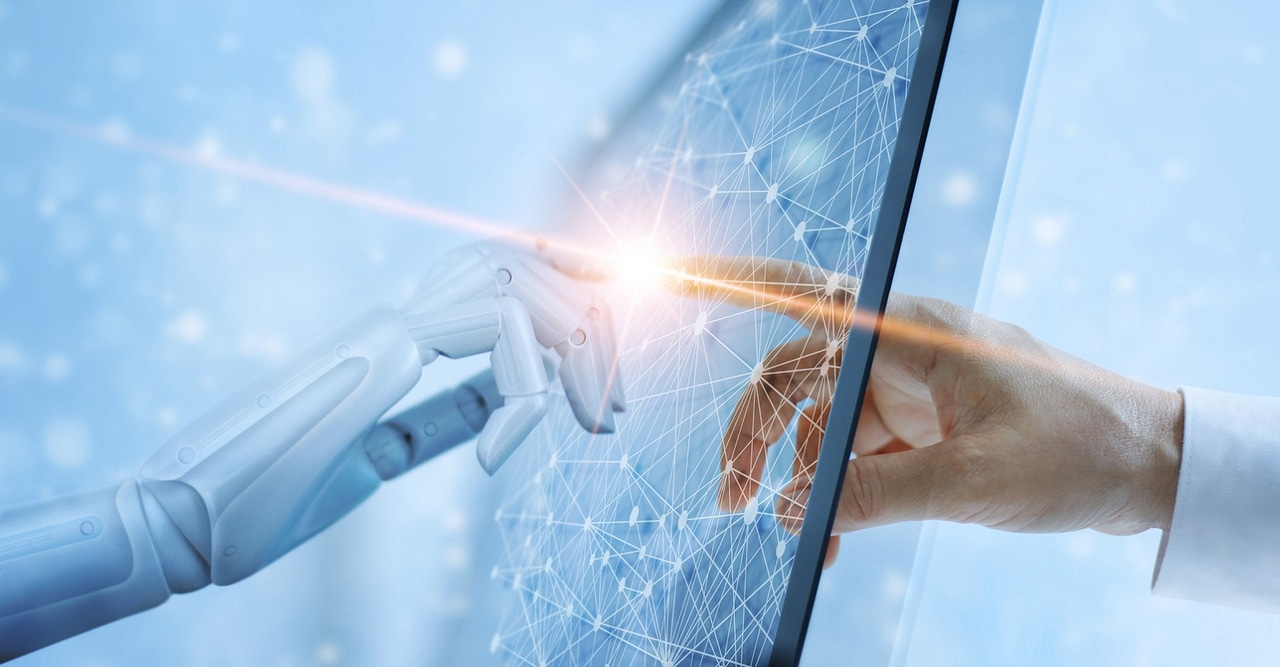
Truly, every year in the past few has been a big one for artificial intelligence and machine learning. As technology becomes both increasingly advanced and increasingly accessible, more parts of our lives and work are touched by AI.
This has both opened up new enterprise opportunities and created new challenges, from chief technology officers with an ever-increasing sphere of responsibility down to individual employees who are concerned about automation and adjusting to new technology in the workplace.
DarwinAI, a Waterloo, Ontario-based AI startup, is well-placed to comment on these shifts –– and on the shifts sure to come in 2020. Sheldon Fernandez, DarwinAI’s CEO, looks back at enterprise AI and ML in the past year and what to expect in the year ahead.
What were the most significant developments, from an enterprise perspective, for artificial intelligence and machine learning in 2019?
I believe one of the most significant developments in 2019 was the increased focus on AI’s "black-box" problem and the amount of discussion, analysis and scholarship (including ours) that emanated as a result.
Moreover, unlike years prior, much of the dialogue had a practical bent to it –– identifying operational complexities and implications, for example –– suggesting that AI initiatives and projects are moving outside the lab for many organizations.
How do those developments set up the enterprise AI space for the coming year? What are you paying especially close attention to?
Explainability will continue to be a hot topic in 2020, but with an emphasis on practical solutions for the enterprise. We will be paying special attention to toolsets that purport to illuminate AI’s black box in a manner that is useful for enterprise organizations –– not least because we will be releasing such a solution ourselves.
Multiple surveys have indicated that enterprises are eager to bring AI into their operations in some way, though execution of that desire is still mixed. Do you think their eagerness is warranted? How can it best be translated into reality, for the average organization, in 2020?
The eagerness is indeed warranted given the significant achievements of "second-wave" AI: incredible image and video recognition capabilities, Natural Language Processing (NLP) advancements and advanced data analytics, etc. In some respects, the situation is not dissimilar from the internet in the 1990s: the vibrations of a transformative technology becoming increasingly acute, with enterprises eager to harness its power.
To translate the promise into reality, organizations should start with "low hanging fruit" and identify simple use cases where AI’s aforementioned capabilities have a demonstrable economic impact. For example, one of our industrial clients is leveraging AI’s perceptual strengths to identify damage on power lines in remote areas using drones. Once identified, they can then send human teams to address the problem. By sending "advanced AI scouts" to survey the damage, they’ve reduced their maintenance costs by orders of magnitude.
Are any particular fields showing notable progress in bringing AI/ML into their operations in meaningful ways? Which sectors have the most potential to make similar moves in the coming year?
Last year, we saw substantial progress in the automotive, aerospace and consumer electronics sectors, all of which leveraged AI/ML in useful ways: autonomous vehicles, conversational intelligence and anomaly detection. In 2020, I suspect we’ll see similar moves in healthcare, financial services and robotic process automation.
There's a lot of excitement about enterprise AI's transformative potential, but is any of the hype unwarranted? Where do you think there's still a lot of work to be done?
As Professor Brian Cantwell Smith articulated eloquently in his recent book ["The Promise of Artificial Intelligence: Reckoning and Judgment"], while AI can do many things extremely well, understanding the richness of the universe –– which comes from authentically engaging "the world as the world" the way children do –– is still far beyond its capabilities.
In other words, AI systems lack true contextual understanding or "meta-knowledge" –– an awareness of the world and themselves within it. For example, while a self-driving car can orient itself on the road, it is completely ignorant of the concept of transportation itself: why humans do it, the efficiencies (or inefficacies) it brings to our lives, the implications to our planet and non-human species, etc.
For this reason, the capabilities of AI will need to be complemented with human wisdom and creativity for the foreseeable future.
Is there anything else of note or that you're watching for 2020?
Though unheralded in mainstream circles, the rise of transformer-based, large-scale language models such as BERT and ROBERTa was a hugely significant development in the realm of NLP [natural language processing], achieving state-of-the-art performance in General Language Understanding Evaluation (GLUE). Considered by many to be the "ImageNet moment" for NLP, numerous teams, including ours, have built on such work for critical tasks as Fake News Detection.
It will be fascinating to see how this new capability is leveraged by the enterprise in 2020.
About the Author(s)
You May Also Like