Reinforcement Learning Gaining Steam in the Enterprise
With its ability to work with controlled environments, reinforcement learning is proving its value in the enterprise.
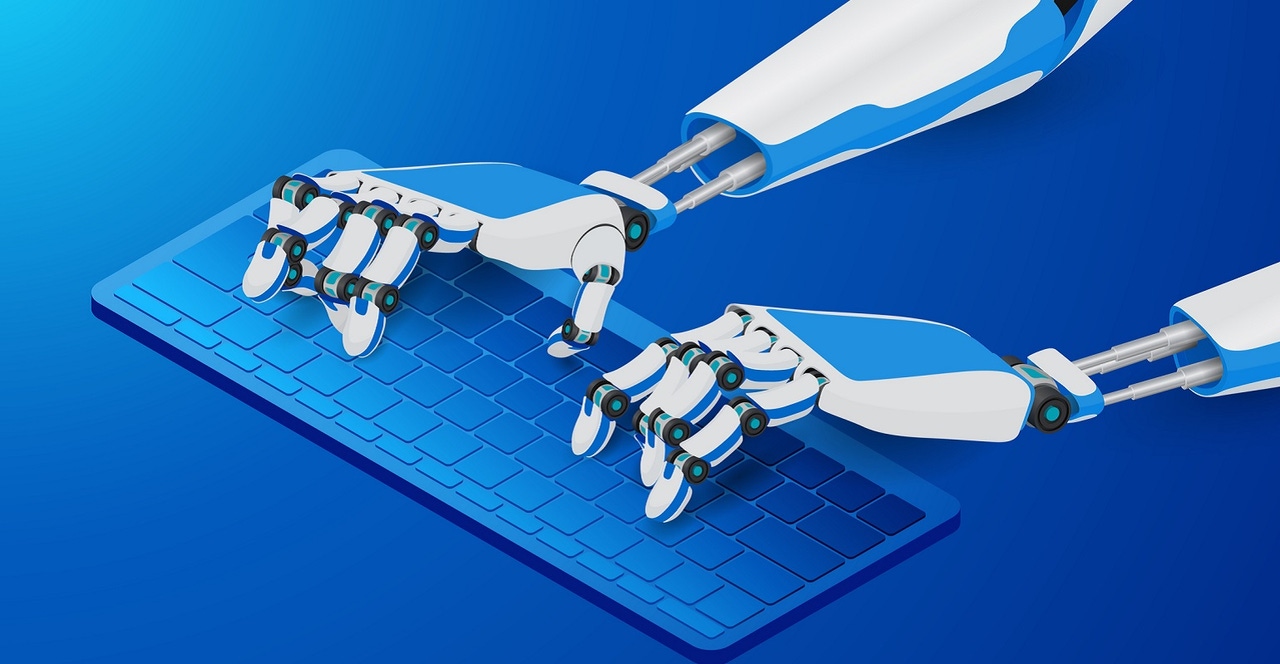
When it comes to machine learning, supervised learning has long been the superstar. But recent advancements and emerging enterprise applications are putting new attention on reinforcement learning.
In an analysis of more than 16,000 artificial intelligence (AI) research papers undertaken by MIT’s Technology Review, reinforcement learning emerged as one of the leading trends in the past few years, following a similar rise of deep learning.
One of three different types of machine learning, along with supervised and unsupervised, reinforcement learning uses a system of rewards and punishment to train models.
Technology Review found that the machine learning type is being mentioned increasingly more often in paper abstracts, but the concept of reinforcement learning has actually been around for a long time, said Vaclav Vincalek, a tech entrepreneur and partner with Future Infinitive.
Surprisingly enough, we have cats to thank for the research concept behind reinforcement learning. Psychologist Edward Thorndike studied behavior in cats and other animals. He found that if you put a cat in a puzzle box and placed a scrap of fish outside, the cat would of course be motivated to escape. In their efforts to get out, the cats would eventually stumble on the lever that released them. In repeating the experiment, the cats figured out that the lever was the trick to their freedom (and the fish) and pressed it increasingly more quickly every time they were put in the box.
Thorndike’s resulting law of effect — that an action that results in a pleasant consequence is likely to be repeated, and one that results in a negative consequence is likely to be stopped — is an idea has been used by AI researchers for decades. What has changed in more recent years is that reinforcement learning is becoming more effective.
That is thanks in part to research done after the unexpected 2016 defeat of the world Go champion by DeepMind’s AlphaGo, which was trained with reinforcement learning. Some of that research is already being applied to enterprise applications.
Reinforcement Learning in the Enterprise
In the enterprise space, Vincalek said, reinforcement learning is currently used in robotics, business strategy planning, telecommunications — “in other words, where the environment is known but the analytical solution is not.”
Some of the areas where reinforcement learning makes sense are clear if you think about what improves with practice when done by humans — for example, driving. Reinforcement learning has been used in AI driving simulations where virtual cars complete a course over and over and over. Theoretically, that approach could be behind the software powering real-world autonomous vehicles following set routes. Mobileye, Google and Uber have said they are testing reinforcement learning for their vehicles.
Reinforcement learning helps companies see which action yields the higher reward over the longest period, Vincalek said. “You’ll find financial companies use reinforcement learning for stock trading, for example,” he said. “It’s also useful in healthcare, with optimization of treatment policies or clinical trials.”
The applications continue to grow. Reinforcement learning can be used to select news briefings to show a client, automate financial trading and select online advertisements, among other potential functions. Reinforcement learning is also used in more general areas that have a place in a variety of industries, Vincalek said. Think of game theory, control theory, operations research, information theory, simulation-based optimization, multi-agent systems, swarm intelligence, statistics and genetic algorithms.
What are the challenges? Reinforcement learning does require significant amounts of data. The technique is also suited to controlled environments, but some environments are uncontrollable and all have unexpected events — a child who runs across the road, for example, or a global pandemic that roils stock markets.
With reinforcement learning, there is significant potential but the movement from simulation to reality will not necessarily be smooth. After all, the consequences with real-life autonomous vehicles are of course potentially more serious than with those that only exist virtually.
About the Author
You May Also Like