How to Safely, Effectively Fill Your Enterprise Data Lake
Here are 10 guiding principles for balancing data democracy with data governance.
May 28, 2020
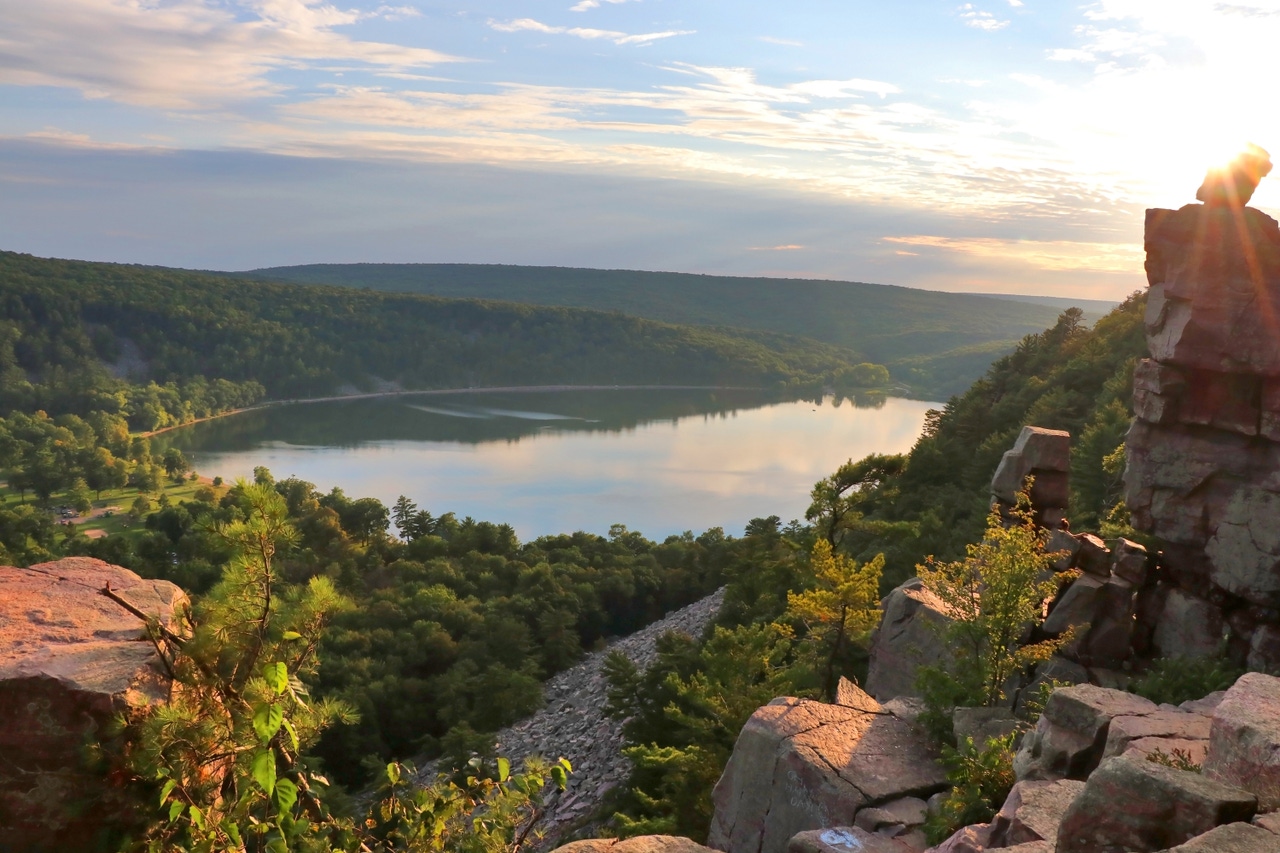
One of the core values of cloud data migration is the ability to share data in a data lake that increases visibility and accessibility to corporate data assets. By democratizing data to a variety of data consumer communities, a cloud-based data lake increases the potential for information monetization. Yet, ungoverned movement of data sets to a shared environment has its risks, which accounts for both the wealth of published guidelines advocating data governance and data stewardship practices as well as the growing number of metadata, data catalog, and data curation tools that are available to help manage and oversee the cloud-based data lake environment.
From a corporate adoption perspective, data lakes are growing in popularity, and this growth is shadowed by a recognized need for discipline. While many of these organizational data lakes are emerging through organic means, it makes sense to take a step back and establish a set of foundational principles to guide the controlled and governed evolution of this critical enterprise information resource. As a start, consider these concepts, which can fuel the development of cloud-based enterprise data lake principles:
Data democracy: The most basic principle of an enterprise data lake is increasing data availability to a wide range of archetypical user communities. The principle of “data democracy” suggests that contributing data sets to the enterprise data lake enables more data consumers to access information that can be used to support the achievement of core business objectives.
Computational independence: The choices of storage platforms for data in the enterprise data lake should not impose any constraints on either the choice or physical situation of the computing platforms that consume and process the data. This principle reinforces the concept of separation of storage from compute.
Platform transparency: The choice of a platform for deploying an enterprise data lake should not create a long-term “vendor lock-in” situation. This principle directs the organization to differentiate between the underlying platform choices and the means by which the data sets are published to the data consumer communities. Platform transparency means employing a hybrid environment and providing the access services that shield the users from the physical deployment.
Findability: As more data assets are moved to the data lake, data consumers may be challenged to find data assets that meet their reporting and analytics needs, or to determine what is contained within each data set. This principle recommends that an enterprise data catalog be used to register assets within the data lake and provide details of structural, object and business-related metadata that can guide data consumers in their choice of data sets.
Data accessibility: Data consumers can easily locate the sources of important data products along with the accompanying services for using those data products. This principle says that data assets that are contributed to the data lake must be accessible by any authorized data consumers.
Data protection: With growing concerns about data privacy and the need to protect against data breaches, it is clear that data protection, security and privacy are of paramount importance to any organization. This principle states that only properly authorized data consumers are granted access to view sensitive data.
Shareability: Curated data products and available data services should be organized in a well-managed registry, categorized according to a well-defined set of taxonomies, and suitable for supporting different development methodologies, including agile and DevOps.
Flexibility: Not all data consumers are able to work with all storage formats. This principle recommends that virtualized layers be made available and imposed on top of contributed data sets that can be tailored to meet the different business needs.
Data quality: Data consumers have an expectation of a level of trust in enterprise data shared through the data lake. Contributed data products must exhibit measurably acceptable levels of data quality data.
Access transparency via data services: Data consumers should not be limited to access via conventional relational database query methods. A robust set of lightweight services should be provided to enable access to data in the data lake.
This is just a starting list. As your organization’s data lake evolves and matures, different stages in the development life cycle will present opportunities for refining the principles so that critical data sets are curated, catalogued and carefully managed. In turn, the enterprise data lake can be protected from becoming a data dump, and instead can provide a means for sharing a healthy inventory of fully described and integrated data assets that can deliver greater business value in a modernized and consolidated way.
About the Author
You May Also Like